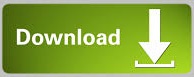
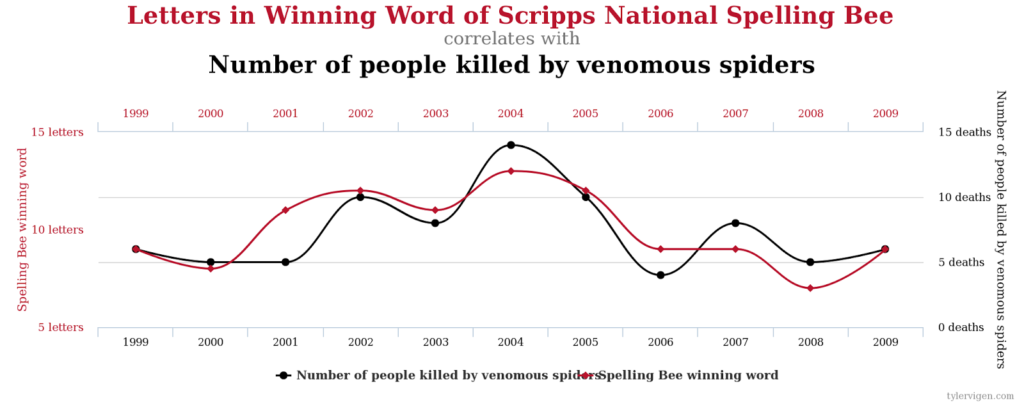
Let's start by talking about correlation in everyday life. (Table containing data on the change in water temperature in a kettle over time) When studying a potential relationship between two variables, it is important to ask yourself the following questions: Does a relationship exist between the two variables? If so, is the relationship positive or negative? What is the strength of this relationship? Is it a strong correlation, a weak correlation, or somewhere in the middle? Correlation can exist between all types of variables, but in statistics, correlation can only be calculated for numeric variables. Correlation plays a big part in data analysis. In the world of data, correlation refers to the existence of a relationship between two variables. This is a very important part of data analysis.
CORRELATION AND CAUSALITY HOW TO
In this video, you will learn how to prove the existence of a relationship, or lack thereof, between two variables. Superstitions like this are a great example of how the brain likes to perceive relationships between two things, even when in reality, no such relationships exist. For example, Ottawa Senators hockey player Bruce Gardiner, was so superstitious, he was convinced the only way he could break the occasional slump in his performance was to dunk his hockey stick in a toilet bowl. When two variables appear to be so closely associated, it can seem that one is dependent on the other. Have you ever noticed the way the human mind really likes patterns? So much so in fact, that the mind will often create patterns. (Image combining a hockey stick and a toilet that equals a Stanley Cup with a question mark) (Diagram of the Steps of the data journey with an emphasis on Step 3 - analyze, model and Step 4 - tell the story)Ĭorrelation and causality fall under the final two steps of the data journey: analysis and modeling, and telling the story. Step 3 and 4: Analyze, model and tell the story This diagram is a visual representation of the data journey, from collecting the data to cleaning, exploring, describing and understanding the data to analyzing the data, and lastly, to communicating with others the story the data tell. The data journey is supported by a foundation of stewardship, metadata, standards and quality.) (Diagram of the Steps of the data journey: Step 1 - define, find, gather Step 2 - explore, clean, describe Step 3 - analyze, model Step 4 - tell the story. Learning goalsīy the end of this video, you will learn the answers to the following questions: What is correlation? How can you measure, quantify or interpret correlation when analyzing your data? What is causality? And finally, what are the differences between the two? Steps of a data journey As a prerequisite, before beginning this video, we highly recommend having already completed our videos titled "What is Data" and "Types of Data".

This video is intended for viewers who wish to gain a basic understanding of correlation and causality. (The Statistics Canada symbol and Canada wordmark appear on screen with the title: "Statistics 101: Correlation and causality") Statistics 101: Correlation and causality
CORRELATION AND CAUSALITY FREE
CORRELATION AND CAUSALITY SOFTWARE
Learners will have the opportunity to apply these methods to example data in R (free statistical software environment).Īt the end of the course, learners should be able to:ġ. Over a period of 5 weeks, you will learn how causal effects are defined, what assumptions about your data and models are necessary, and how to implement and interpret some popular statistical methods. We have all heard the phrase “correlation does not equal causation.” What, then, does equal causation? This course aims to answer that question and more!
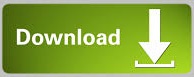